Updated: June 6, 2024- 6 min read
Consumer-grade generative AI has undoubtedly made waves, captivating users with chatbots that can mimic human intelligence and solve hundreds of use cases. The technology holds promise for businesses, too. For Product teams, one allure is GenAI’s potential to deliver in-app self-service analytics to their customers. There is also the pull of delivering hyper-personalized user experiences and enabling new ways to monetize data.
According to McKinsey, GenAI could bring economic benefits to the tune of $4.4 trillion to the global economy, annually.
Deploying generative AI for business is no longer a question. The question is – where you deploy it, and how you deploy it so that it delivers value without the risks associated with consumer-grade GenAI.
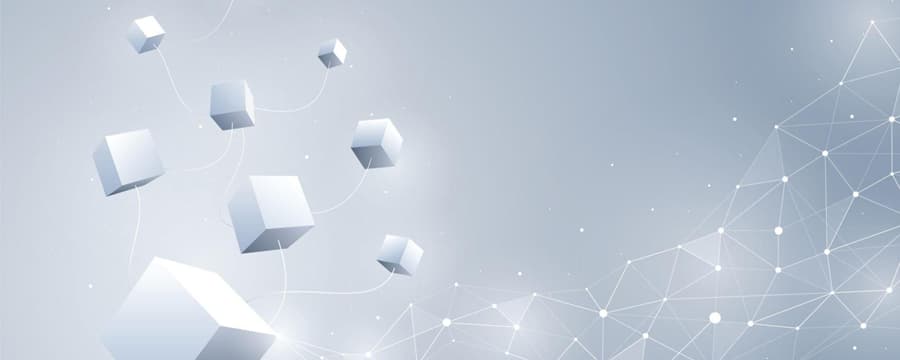
The Question of Where: Most Profitable Use Cases of GenAI for Product Teams
According to a recent Forrester report, embedding GenAI in first-party and third-party apps will be more useful than launching standalone apps.
For example, customer-facing apps can leverage GenAI to automate repetitive tasks, such as gathering key insights from complex financial reports, or automatically building dashboards based on underlying datasets. Imagine generative AI embedded in finance software or an HR application, allowing employees to prompt an AI bot for quarterly reports and hiring trends.
Similarly, SaaS companies can offer embedded GenAI to their customers for more value addition. For instance, a SaaS billing platform with embedded GenAI gives customers accurate predictions on subscription renewal. It automatically highlights accounts most likely to leave based on historical usage patterns, thereby helping companies take action to reduce their attrition rate.
Likewise, a SaaS marketing platform can use embedded GenAI to suggest optimized social media strategies based on trends, user interactions, and historical campaign performance.
When deciding whether a GenAI deployment is worth it or not, consider these two factors:
1. Low-Risk Scenarios
Instead of trying to replace entire workflows with generative AI, use it to enhance existing processes. See where it can be deployed with ease and minimum risk. For example, integrating it in text-heavy and data-heavy tasks, such as creating data dashboards.
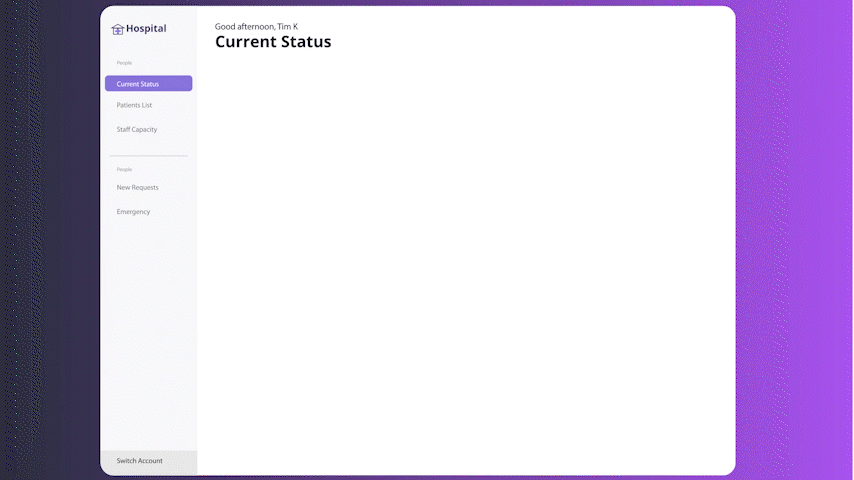
2. High value impact
Ensure that the integration of generative AI aligns with broader business objectives. It should contribute to the overall strategy rather than being a standalone initiative. Positive impacts on Time-to-Market, Cost Savings, and Operational Efficiency can be benchmarks for deciding whether a particular deployment is worth the effort. Pick scalable and sustainable use cases. Sustainable use cases are those that can endure over time. They don't just provide immediate benefits but are designed to evolve and adapt to changing business landscapes and technological advancements.
There are more than a few obvious use cases of GenAI for business. However, using GenAI for business takes much more than firing up ChatGPT (or your favorite AI tool).
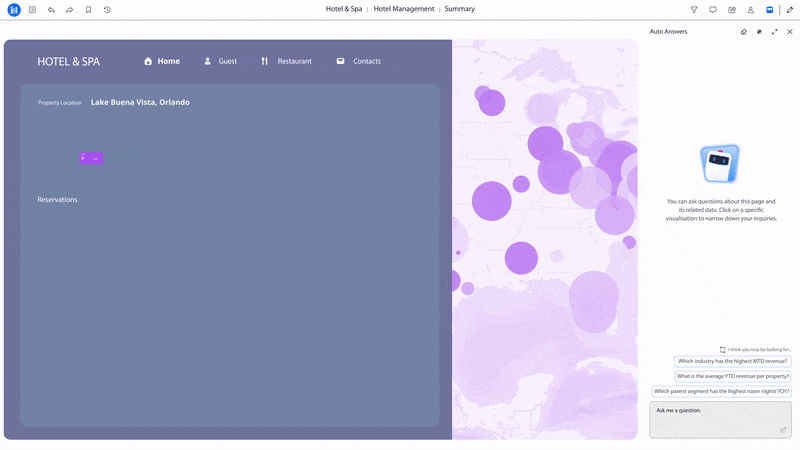
The Question of How: Practical Considerations When Deploying GenAI for Businesses
1. The Critical Role of Domain-Specific and Real-Time Data
The most straightforward applications of GenAI involve knowledge work and information retrieval. Imagine empowering your customers with an AI chatbot that enables them to ask about their most valuable clients or your best-selling products. This shift in how we access business-critical information is poised to automate tasks, such as ad-hoc analytics and dashboard design.
However, there is a caveat – true business readiness demands contextualized and current data. Imagine a scenario where a SaaS company is deploying generative AI to provide users with personalized recommendations, assistance in navigating through complex workflows, and insights into optimizing their usage of the software. For this to be effective, the GenAI must not only understand the broader landscape of SaaS solutions but also deliver responses tailored to the specific features and intricacies of the company's software suite.
It also requires real-time data about the current state of the software, recent feature updates, and user-specific preferences. The challenge lies not just in acquiring and integrating SaaS-specific data but also in ensuring that this data is consistently updated and relevant at the precise moment users engage with the AI component.
2. Secure and Trusted Access: Data Governance
While real-time access to contextualized data is crucial, it must be secure and trusted. Imagine you have customers in a multi-tenant environment, sharing resources with other businesses. Controlled access to business data is crucial in such cases.
A robust governance framework is foundational for ensuring data security and trustworthiness. This involves defining clear policies, procedures, and standards for data access, usage, and storage.
Assigning accountability for data access and usage helps create a structured and responsible approach to handling sensitive information.
Factors of data sovereignty and compliance with regional regulations also need to be taken into account, as much for staying clear of legal troubles as for ensuring consumer trust. Implementing access controls through role-based access, encryption, and authentication mechanisms minimizes the risk of unauthorized use or data breaches. Regular audits and monitoring of access logs further contribute to maintaining a secure environment.
3. The Need for Consolidated Business Logic
ChatGPT, and other Large Language Models (LLMs) – the foundational basis of GenAI – are non-deterministic. Meaning, they do not produce the same output for a given input every time. In other words, if you provide the same prompt to ChatGPT on two separate occasions, the chatbot may generate different outputs each time. The non-deterministic nature is useful in creative tasks, such as writing marketing copy or generating images. However, it is not desirable for other business tasks, such as analyzing data, where consistency and accuracy are paramount.
Imagine you are an insurance company that’s delivering an AI-enabled risk-assessment product to businesses and government agencies. The product is AI-enabled, which means users can prompt the AI engine to generate risk reports based on specific criteria. In this case, you will want reports that are 100% accurate and consistent in their outlook. Any inconsistency will hamper the users’ confidence.
To mitigate the non-deterministic nature of GenAI, it needs a robust layer of consolidated business logic that sits like a bridge between data sources and the AI component. By applying business logic to the input prompts and GenAI outputs, this layer ensures that the information presented to the end-users is accurate and consistent with their expectations.
Business Tasks Need Business-Grade GenAI
Consumer-grade GenAI tools are not cut out for business deployment. One, they do not have built-in data governance mechanisms. ChatGPT, for example, stores user data for training its algorithms. And two, they are trained on all the data that exists on the internet. As we have seen above, business use cases need algorithms that are trained on domain-specific contextual data to deliver real value.
In steering their organizations toward the future, Product teams require more than a passing familiarity with GenAI; they need a business-grade solution that encapsulates reliability, security, and strategic alignment. Business-grade generative AI ensures that the promises of innovation translate into sustainable value. It becomes the conduit through which organizations can not only embrace the transformative power of AI but do so with a keen eye on scalability, security, and long-term adaptability. As Product teams champion the integration of GenAI within their product landscapes, they set the stage for a future where innovation is not just a buzzword but a tangible force shaping the success of businesses in a rapidly evolving digital era.
Updated: June 6, 2024